104: Low-code integration using AI
Introduction
Data mapping is among the most important design steps in building an integration flow. This design-time task is usually the most tedious, time-consuming, complex, and error-prone because it requires deep understanding of all the data fields on the source and target systems. Building a successful data mapping also requires business analysis, domain expertise, and technical knowledge on both source and target systems.
Our goal in creating Mapping Assist was to simplify and automate data mapping. And when coupled with the extensive list of pre-built, security rich connectors, it can accelerate the delivery of value to the business through integration.
ACME Retail has acquired another business and needs to synchronize the contacts between two CRM applications. In this demo, we assist ACME using AI and low-code integration, synchronizing contact information between the Salesforce and Insightly CRM systems. We will recognize and automatically match fields with high confidence levels. We will also recommend and assist with other fields where there is lower confidence. And we will generate a transformation across the fields when the field formats are not the same. As you continue to use the Mapping Assist capability it learns from previously accepted matches to raise confidence levels in future mapping efforts.
Using AI-powered mapping, we can reduce errors and speed integration development.
In the demo, we will execute the following steps:
• Access the Cloud Pak for Integration environment
• Create and implement an API-enabled flow to map the fields for ‘contact’ between Salesforce and Insightly CRM
• Auto-map fields wherever the matching confidence is above 80%
• Generate a data transformation when the field formats do not match
• Execute the flow to see the results
Let’s get started!
1 - Accessing the environment
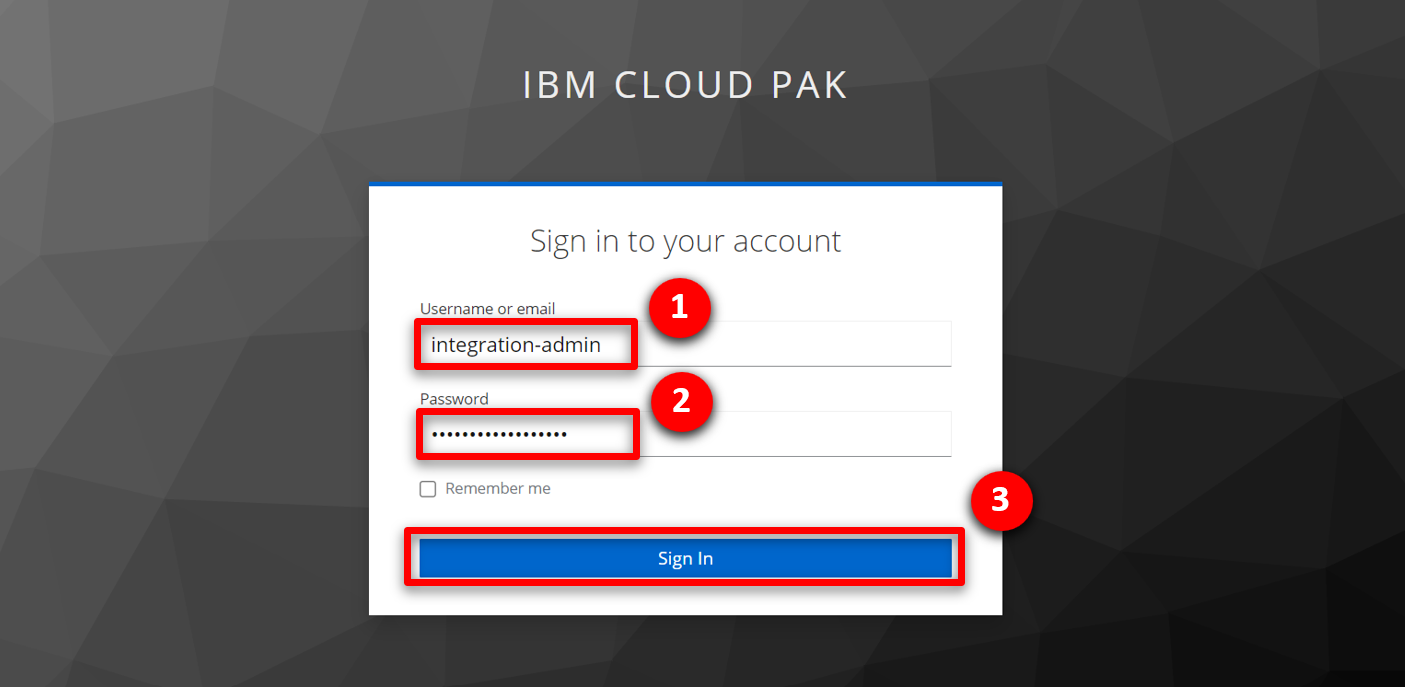
In this demo, we’ll use the App Connect Designer’s Mapping Assist capability to speed the transformation of complex data formats used by cloud applications, such as Salesforce and Insightly. Let’s open our App Connect Designer.
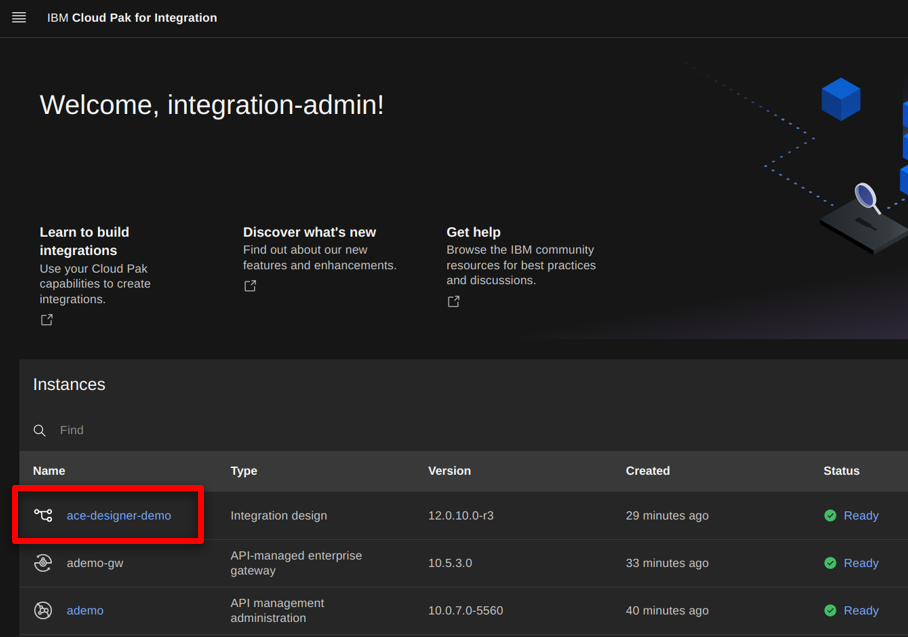
2 - Creating the flow
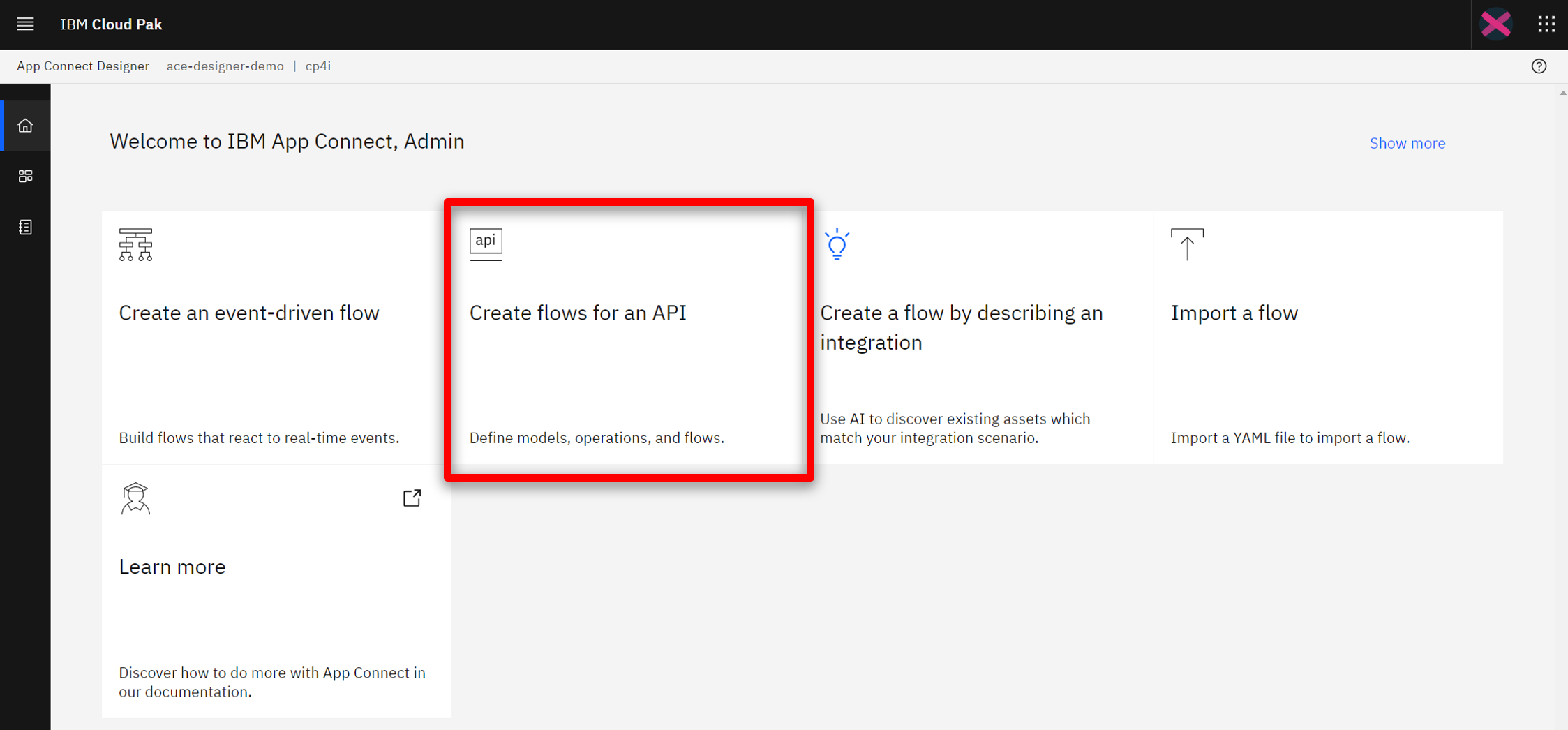
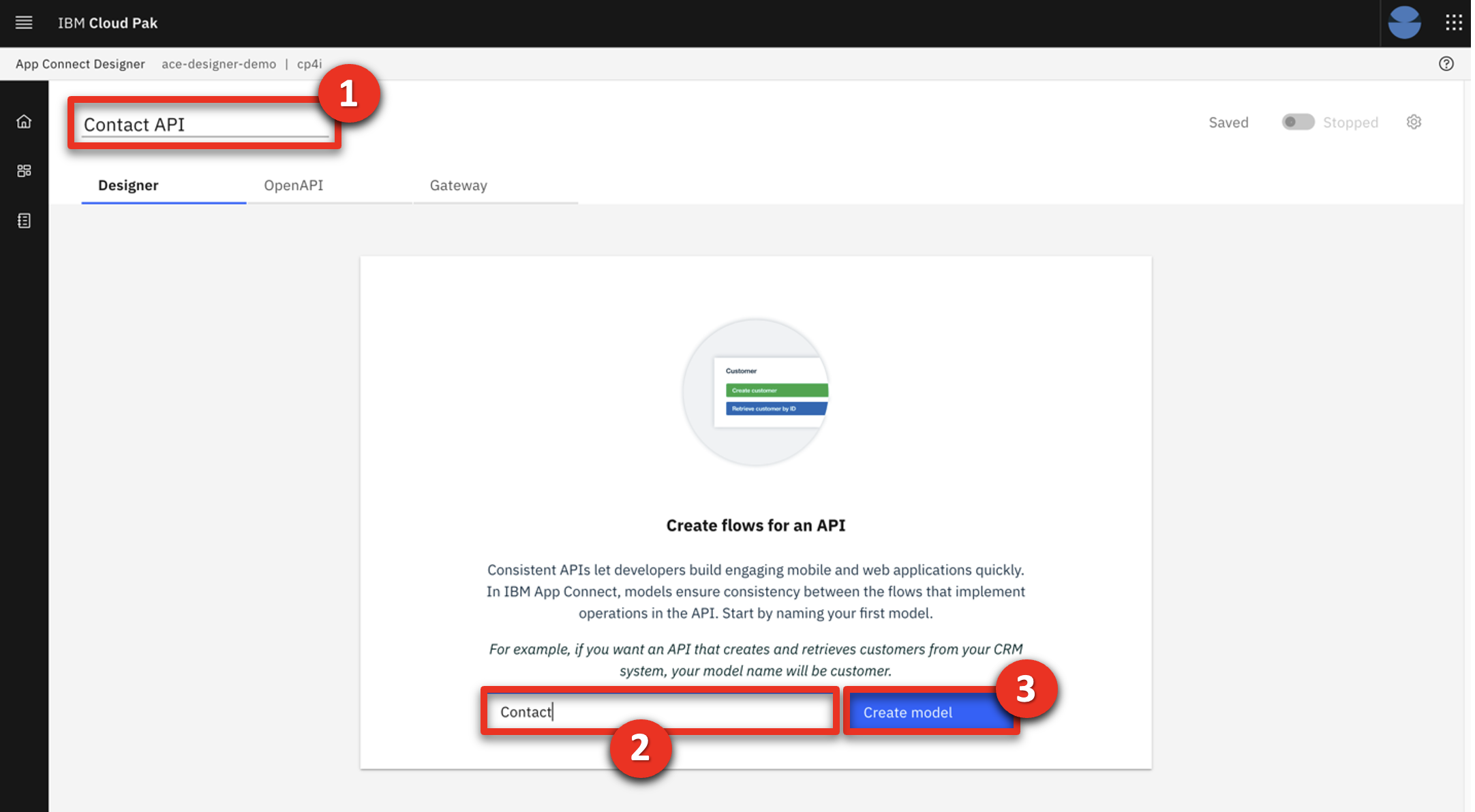
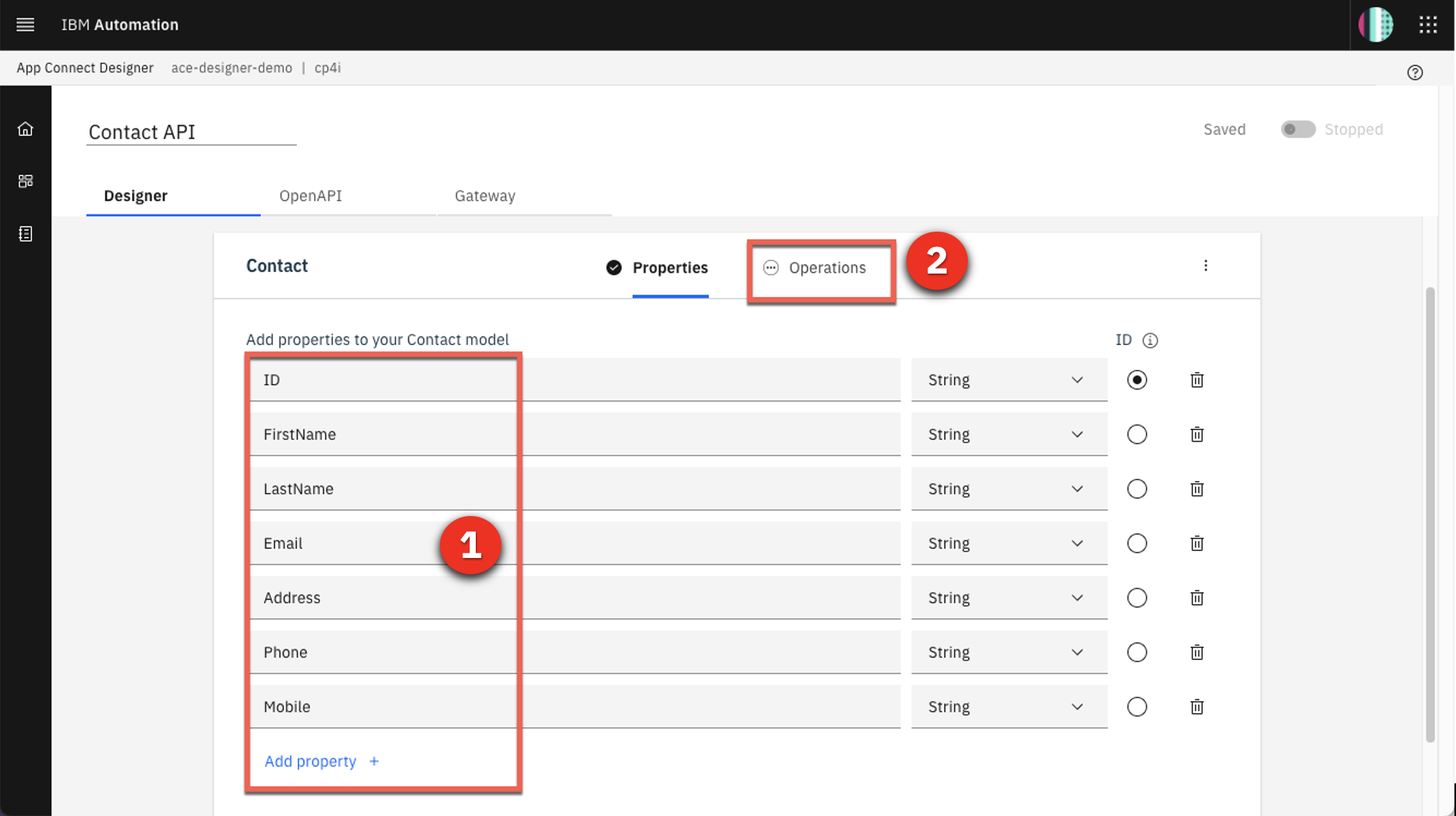
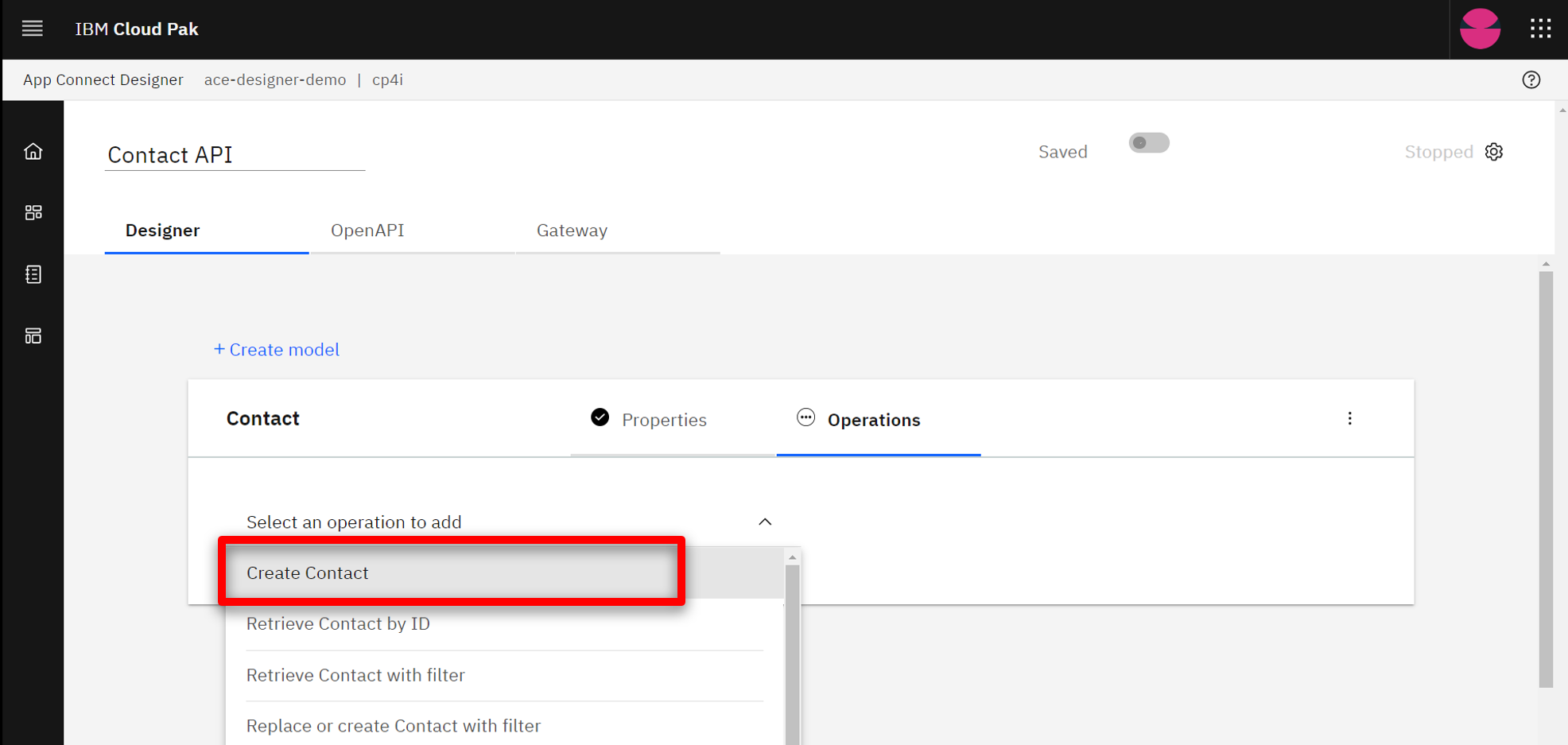
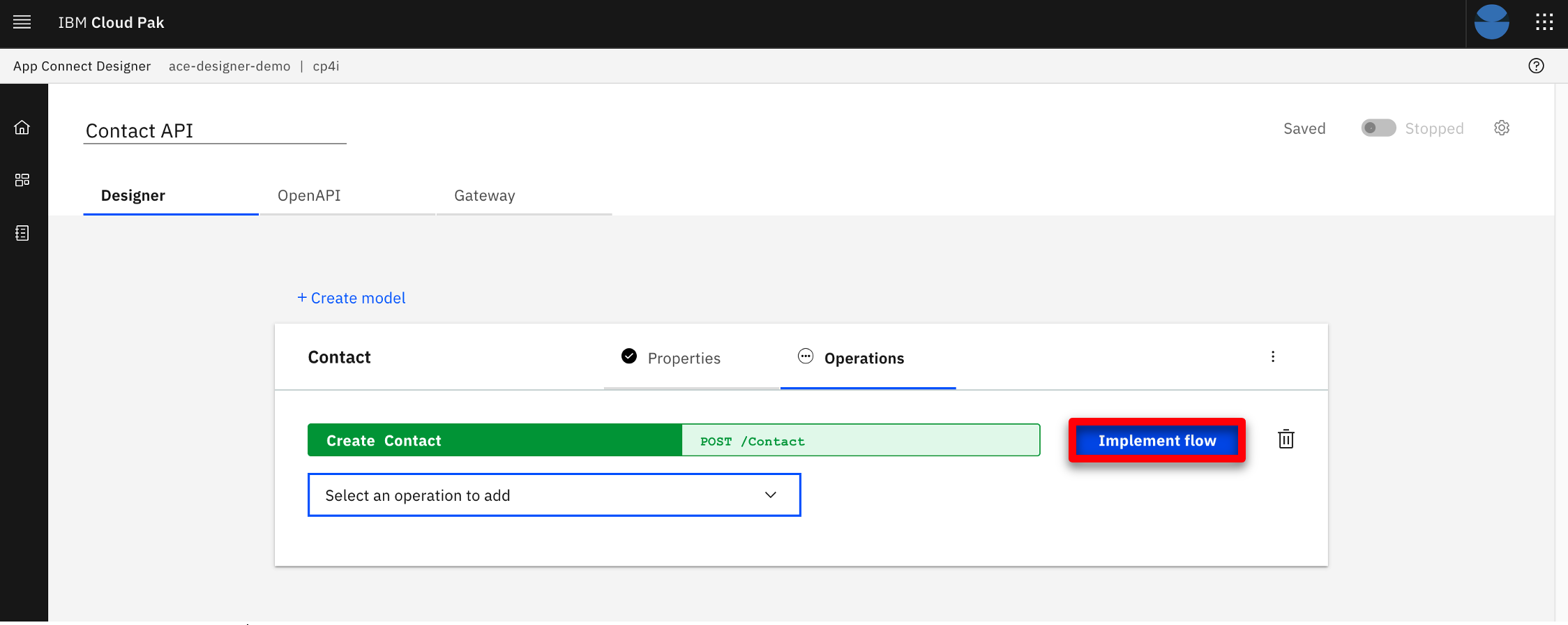
3 - Implementing the flow
Before we add to our flow, let’s adjust the response that is returned when the flow is called. This response serves to close the API flow and is a required construct. Because there is no data needed by the caller of the API flow (but a response is required), we can have a very simple response. We will add the ID to the response.
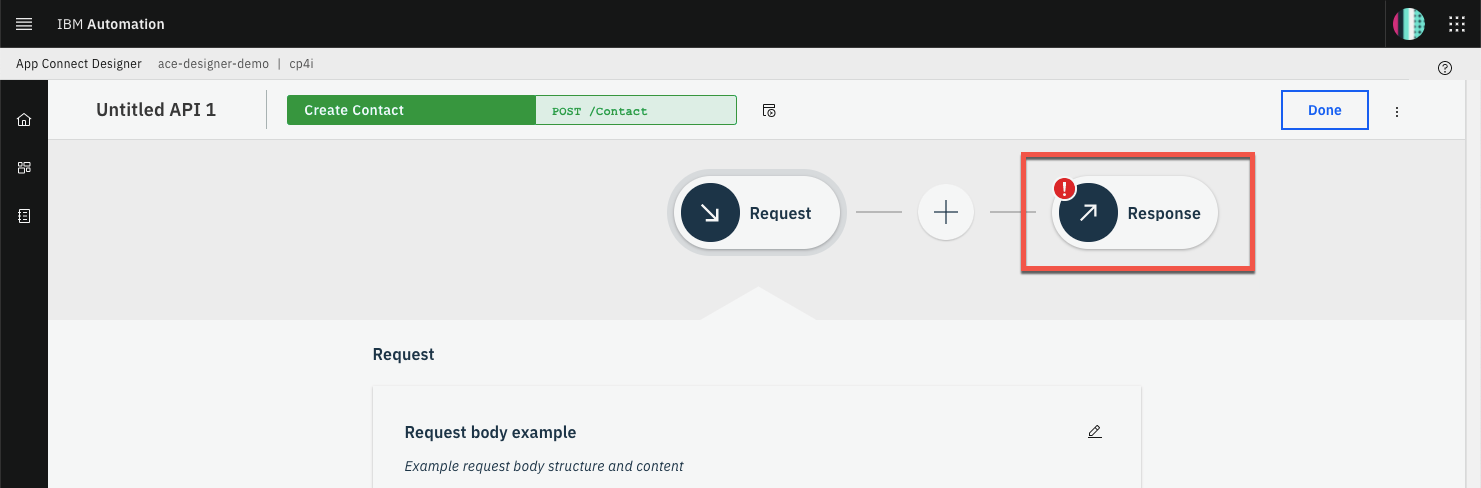
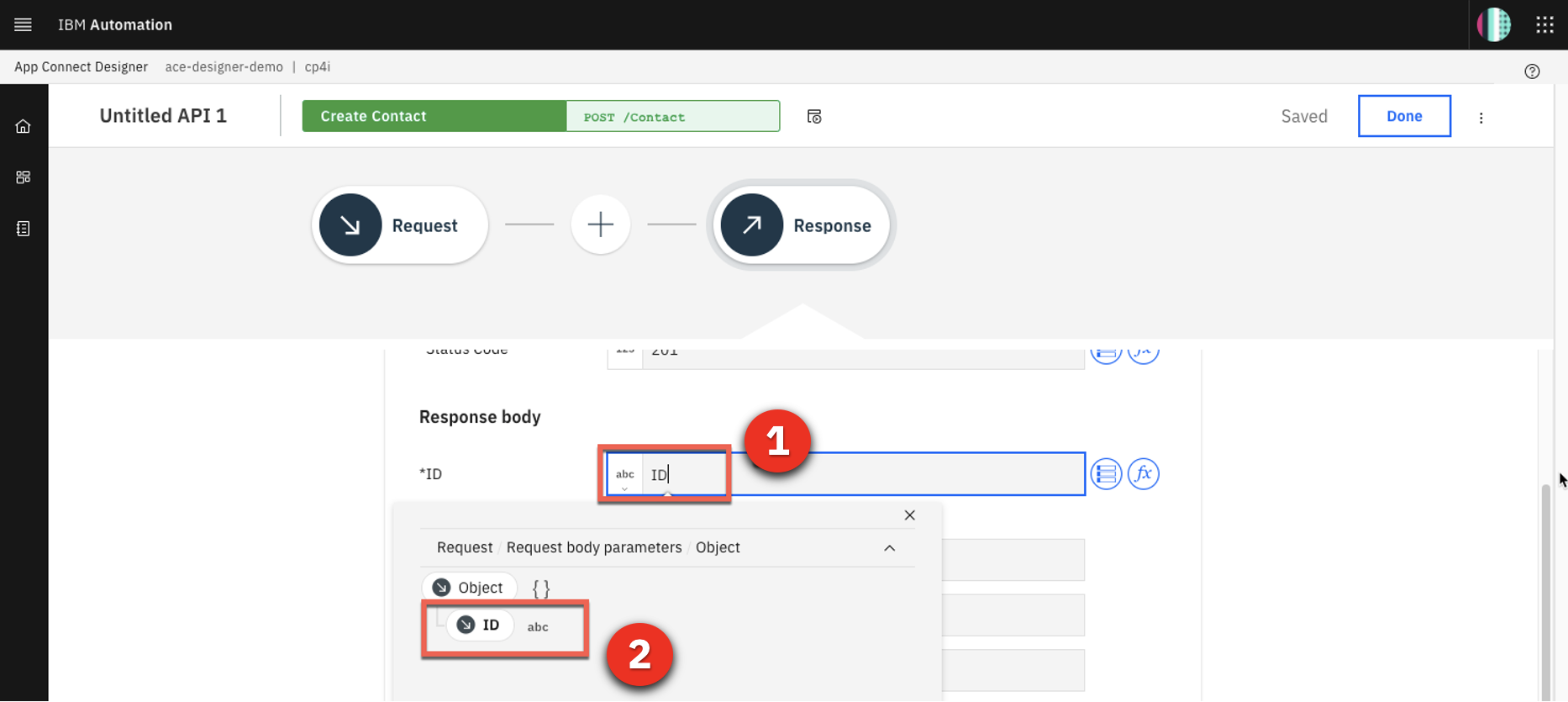
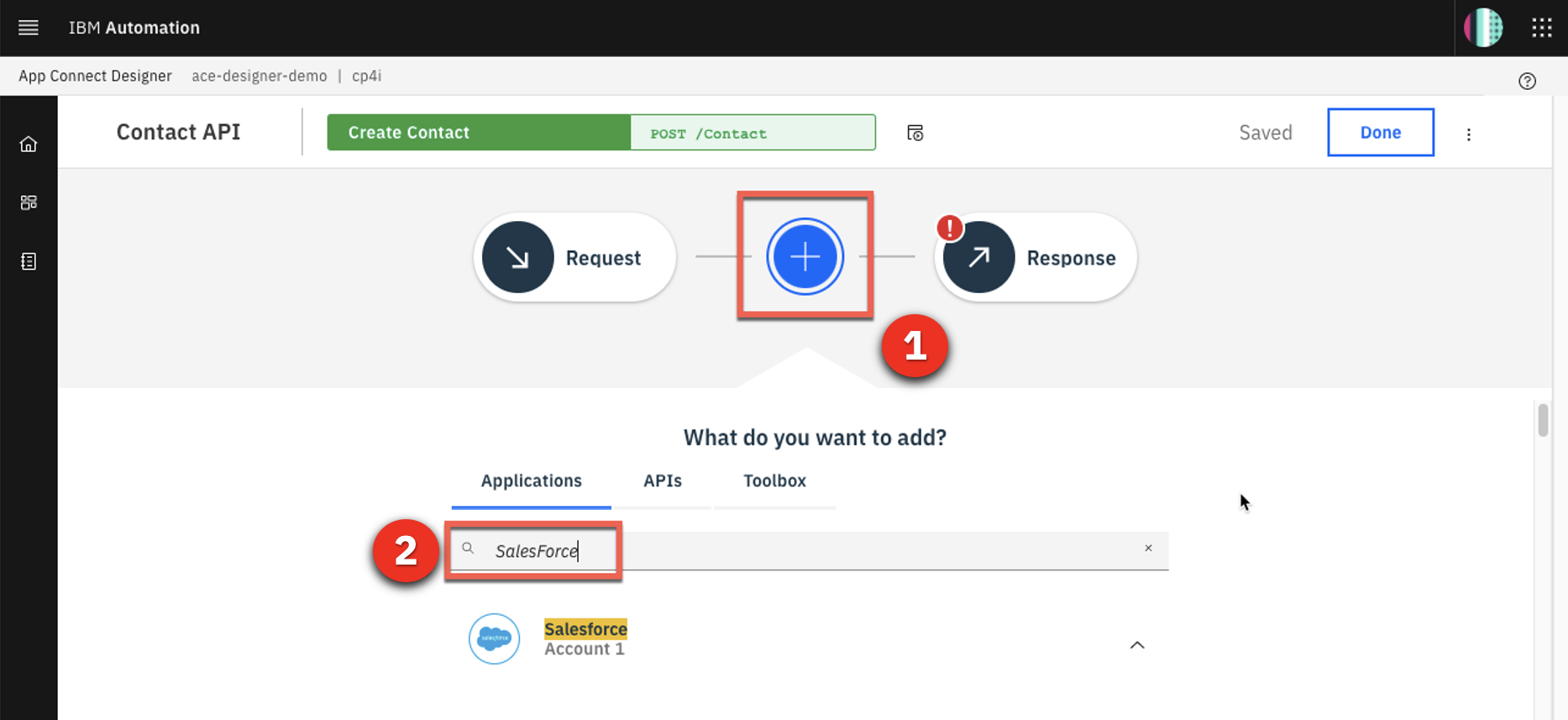
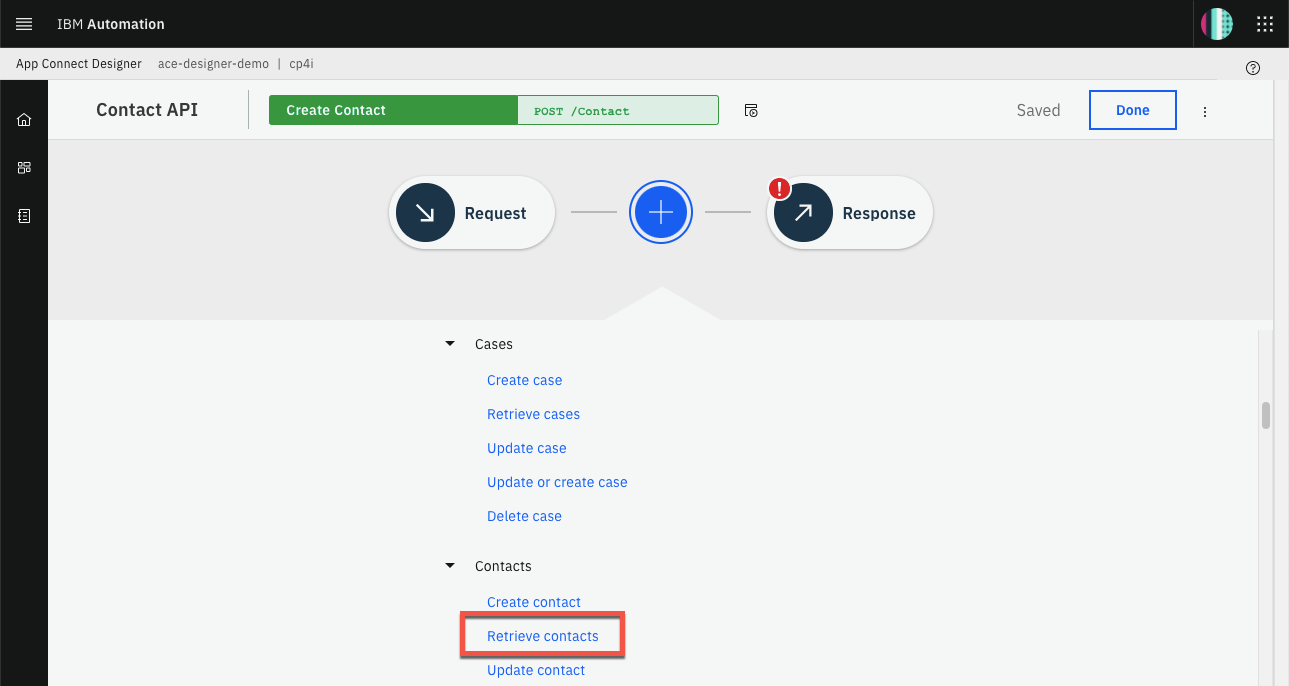
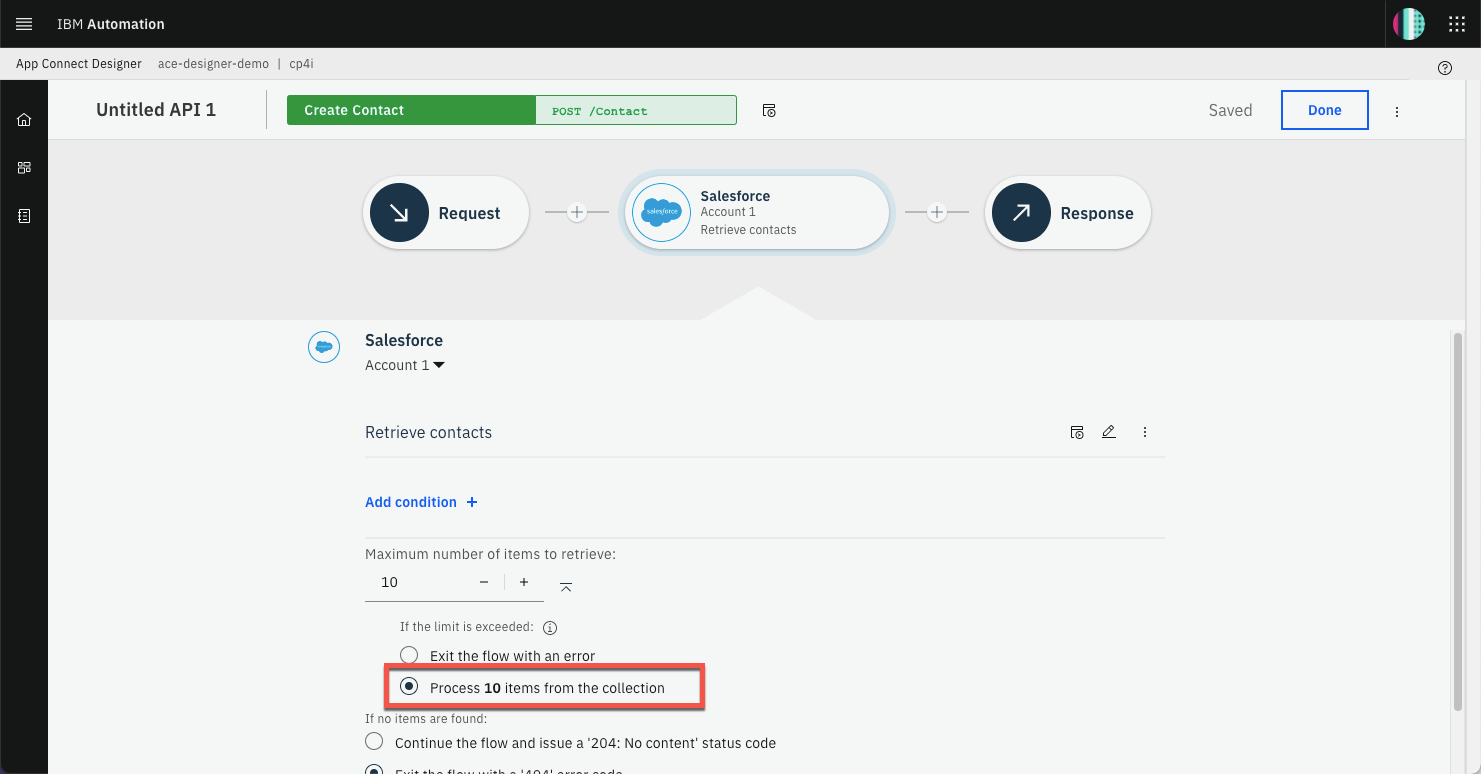
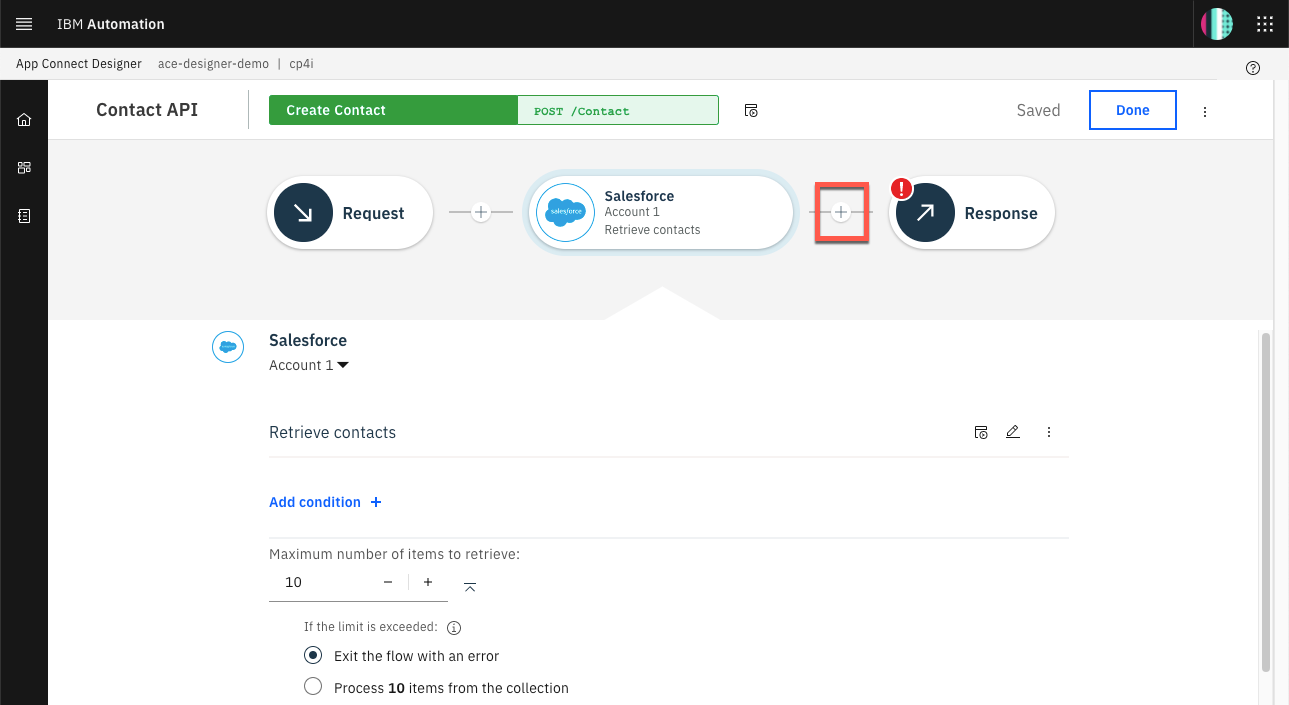
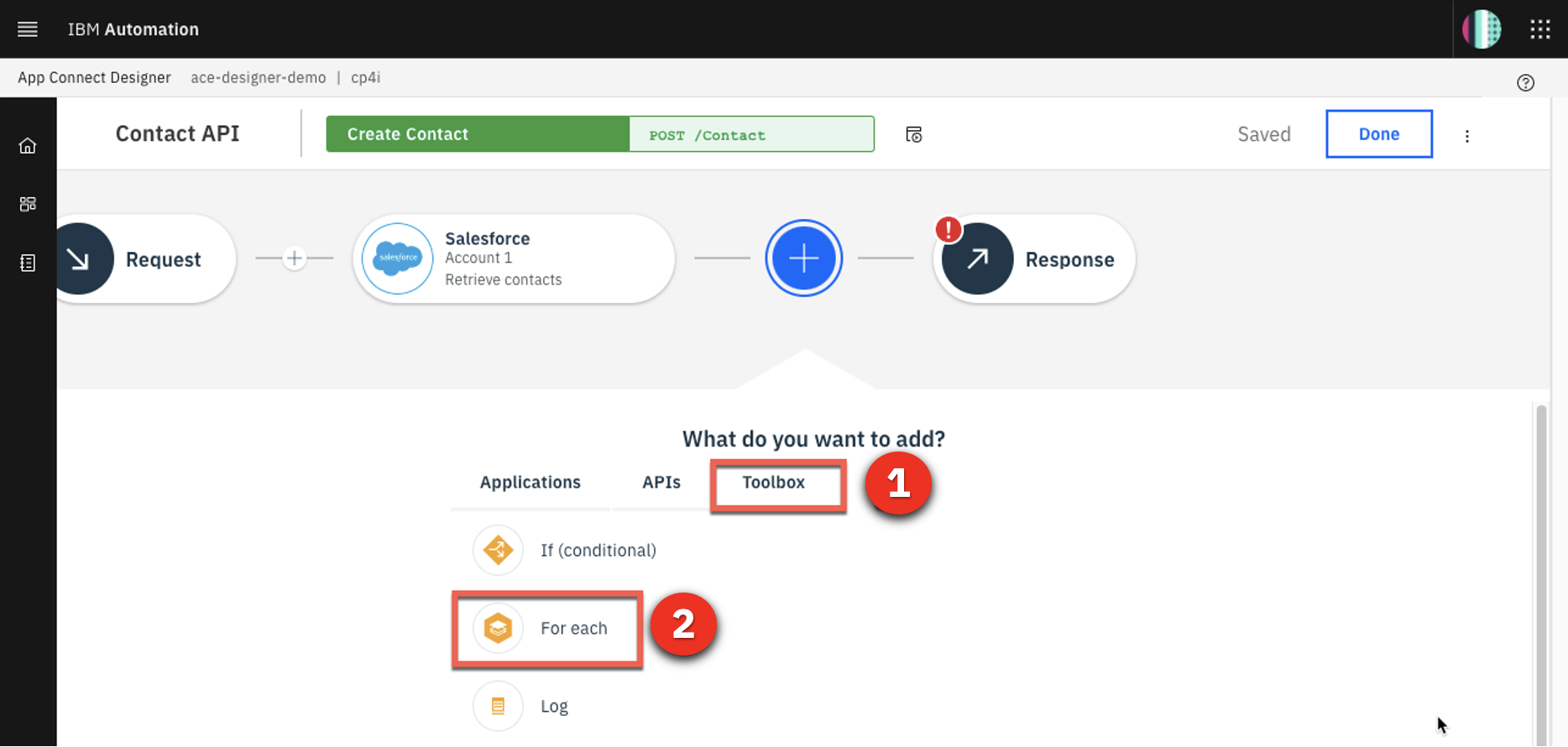
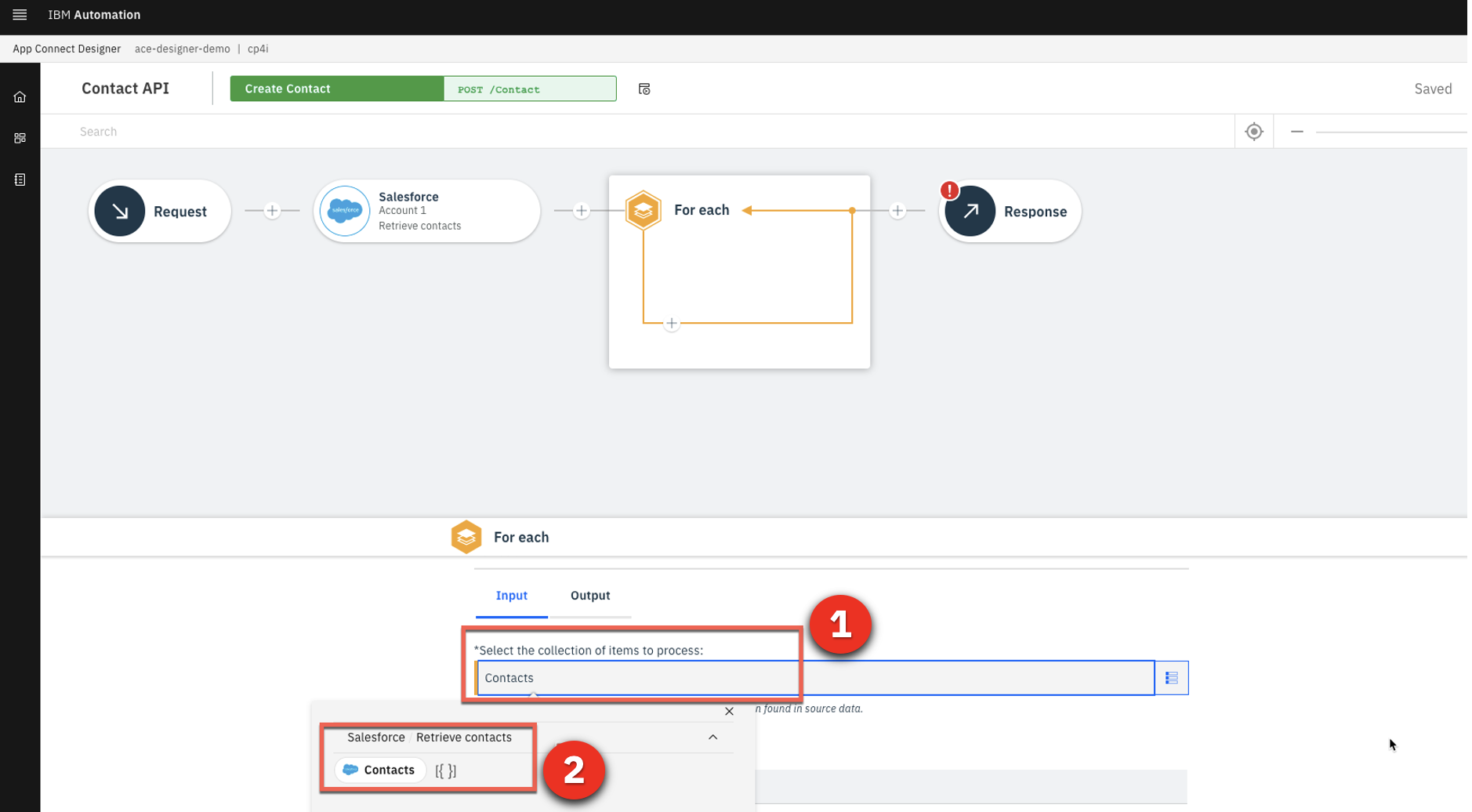
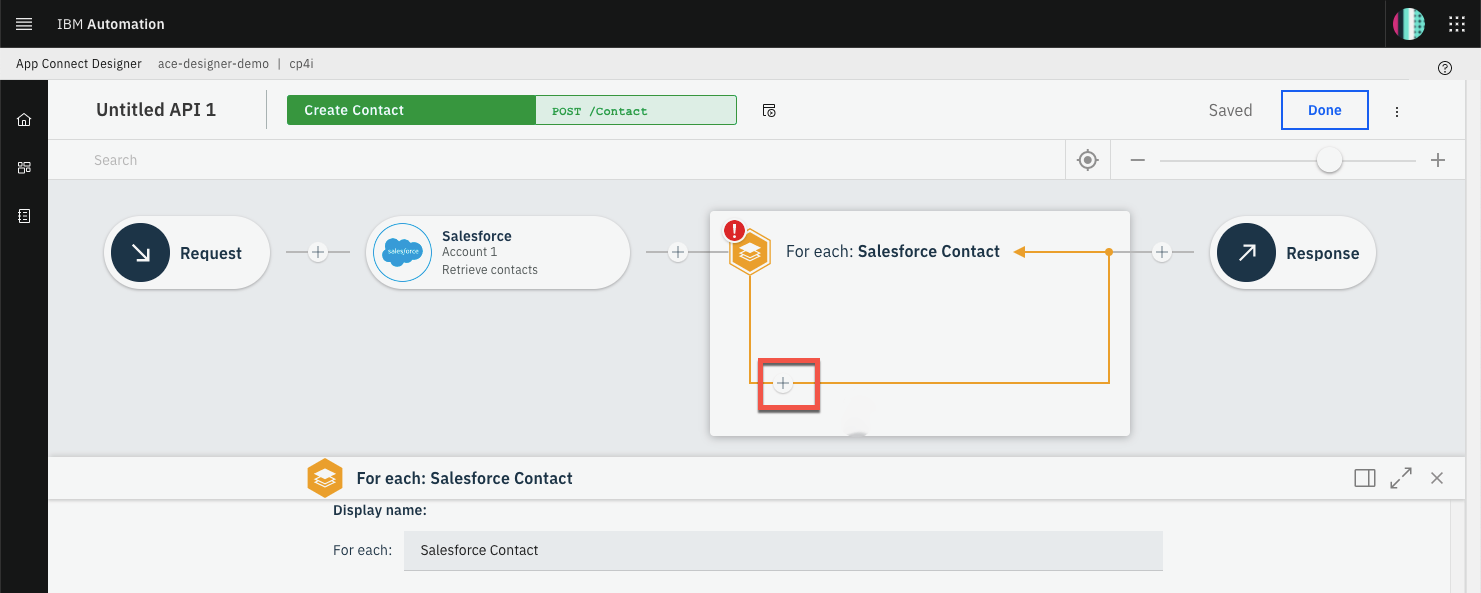
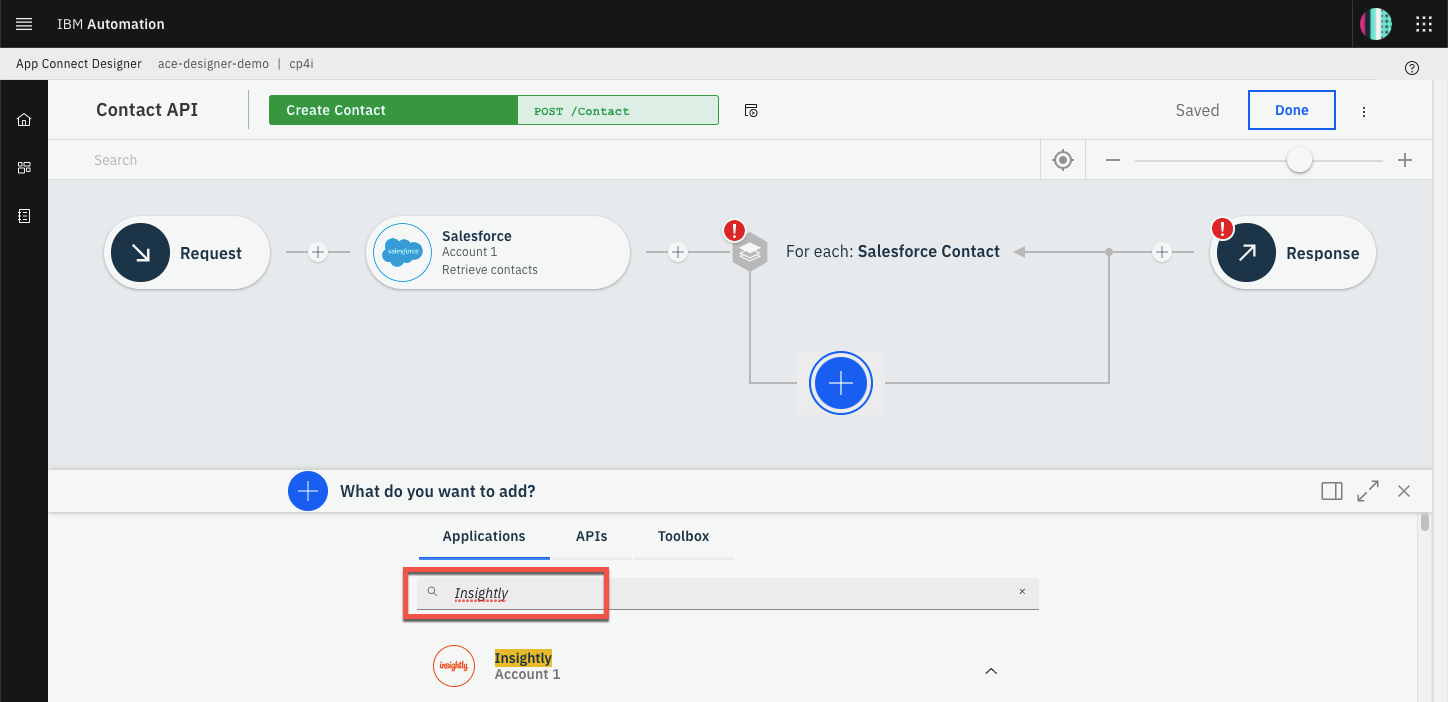
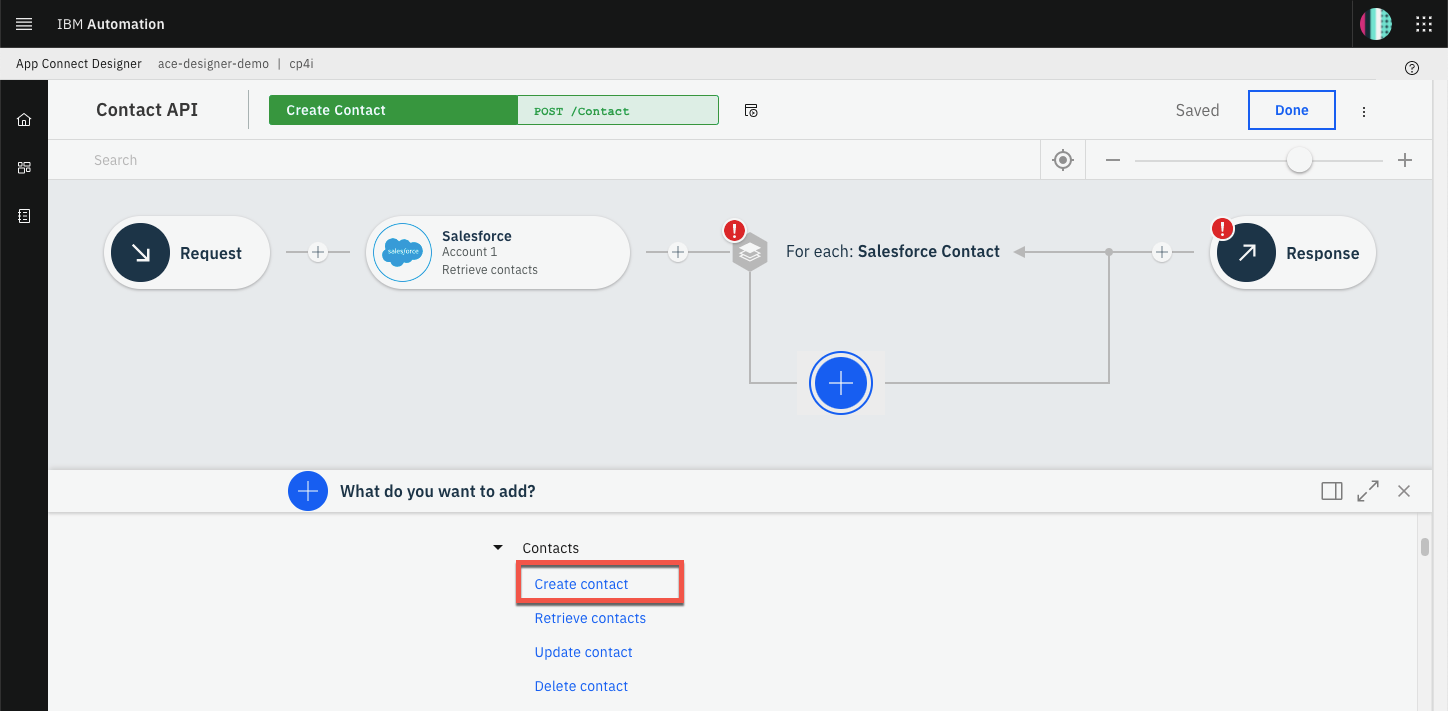
4 - Using the Mapping Assist feature
With Mapping Assist, you don’t need to type all of these mappings. We’ll use AI to simplify our work! Once Insightly’s ‘Create Contact’ node is added, mapping suggestions are automatically generated. The best possible matches (top suggestions) are presented, which can be automatically inserted into fields with a single click. These top suggestions have an 80% (or higher) confidence score, and the count (20+ suggestions) identifies the total number of fields that will be populated with mappings.
The exact number of suggestions may vary.
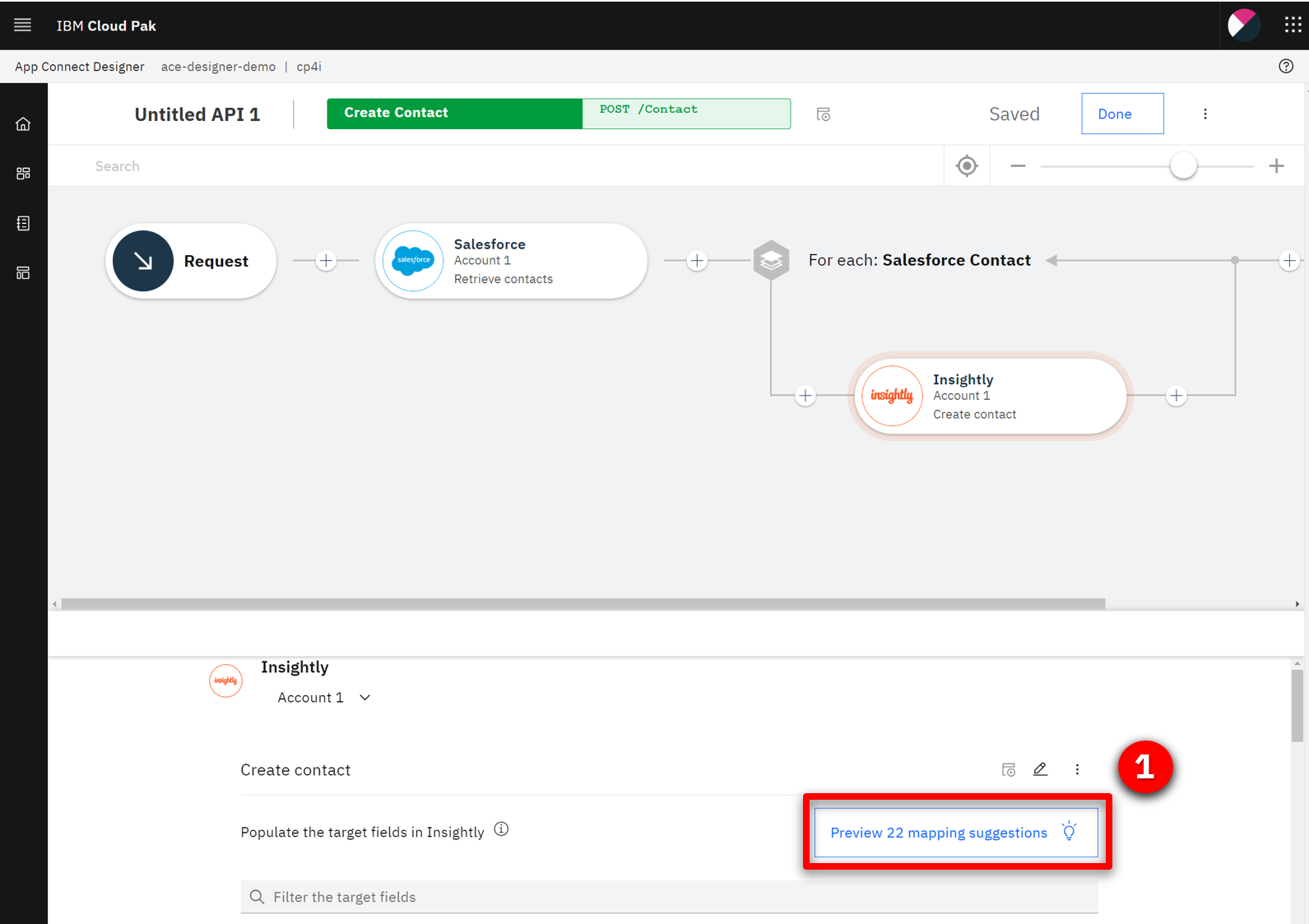
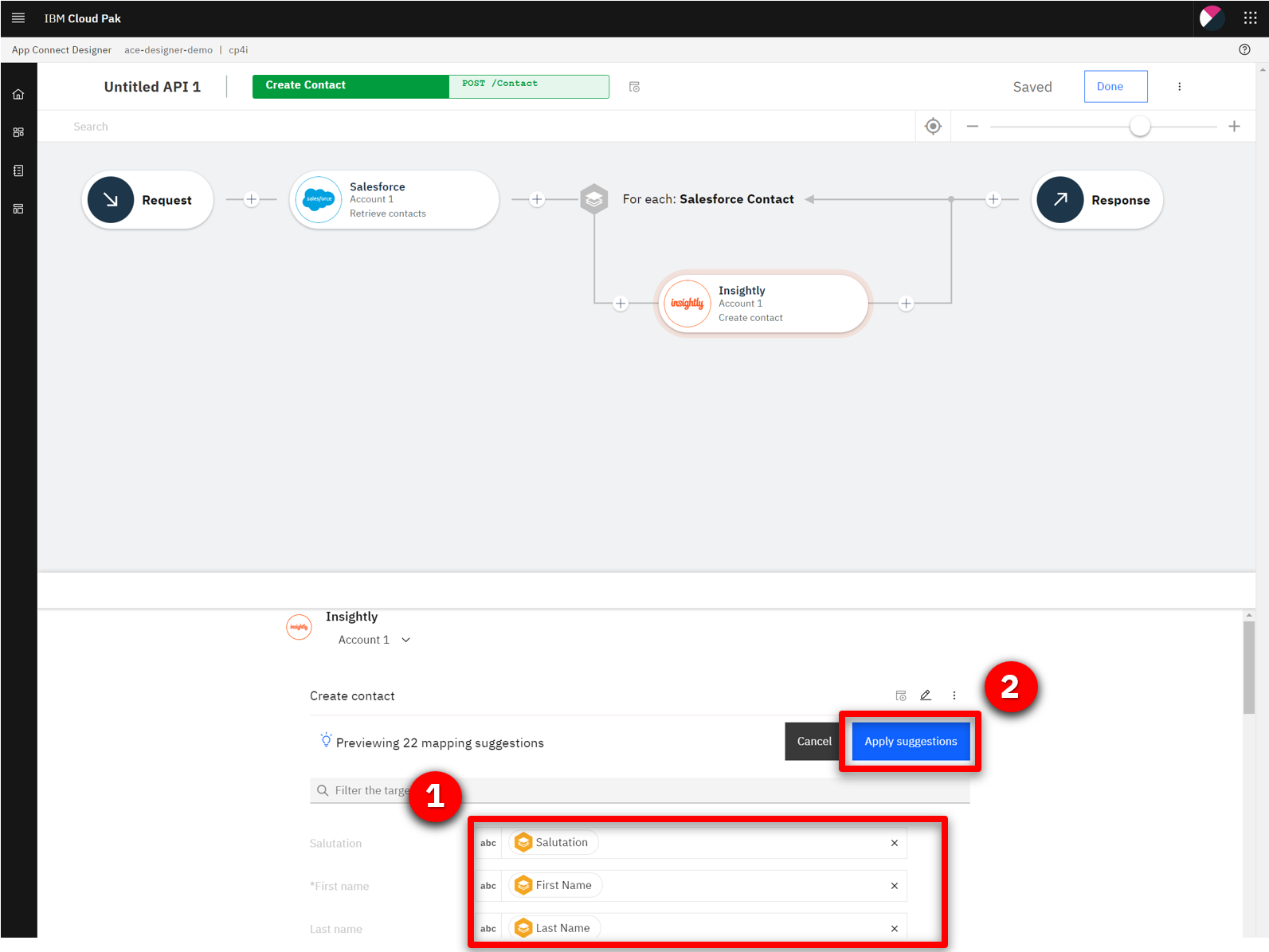
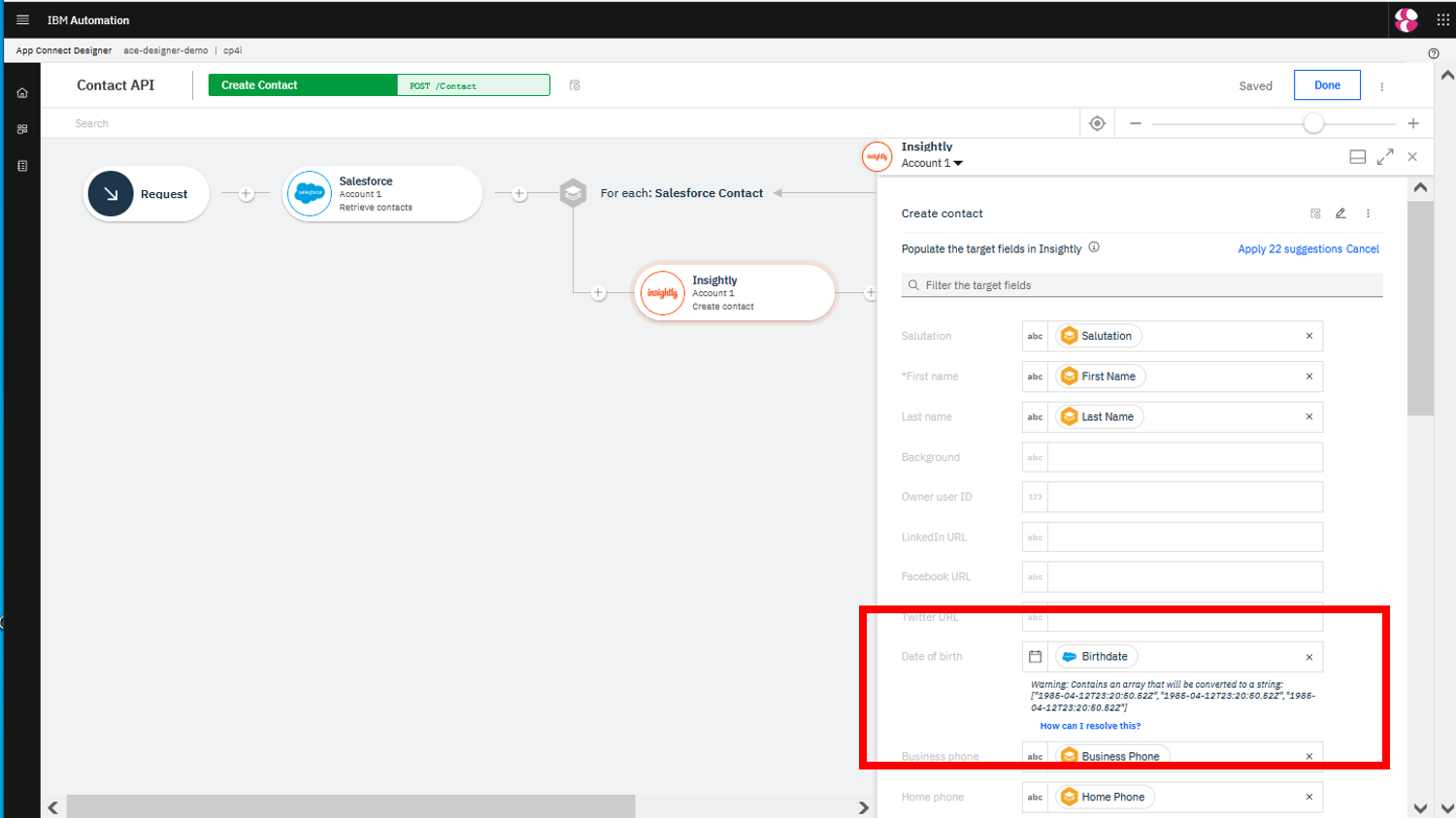
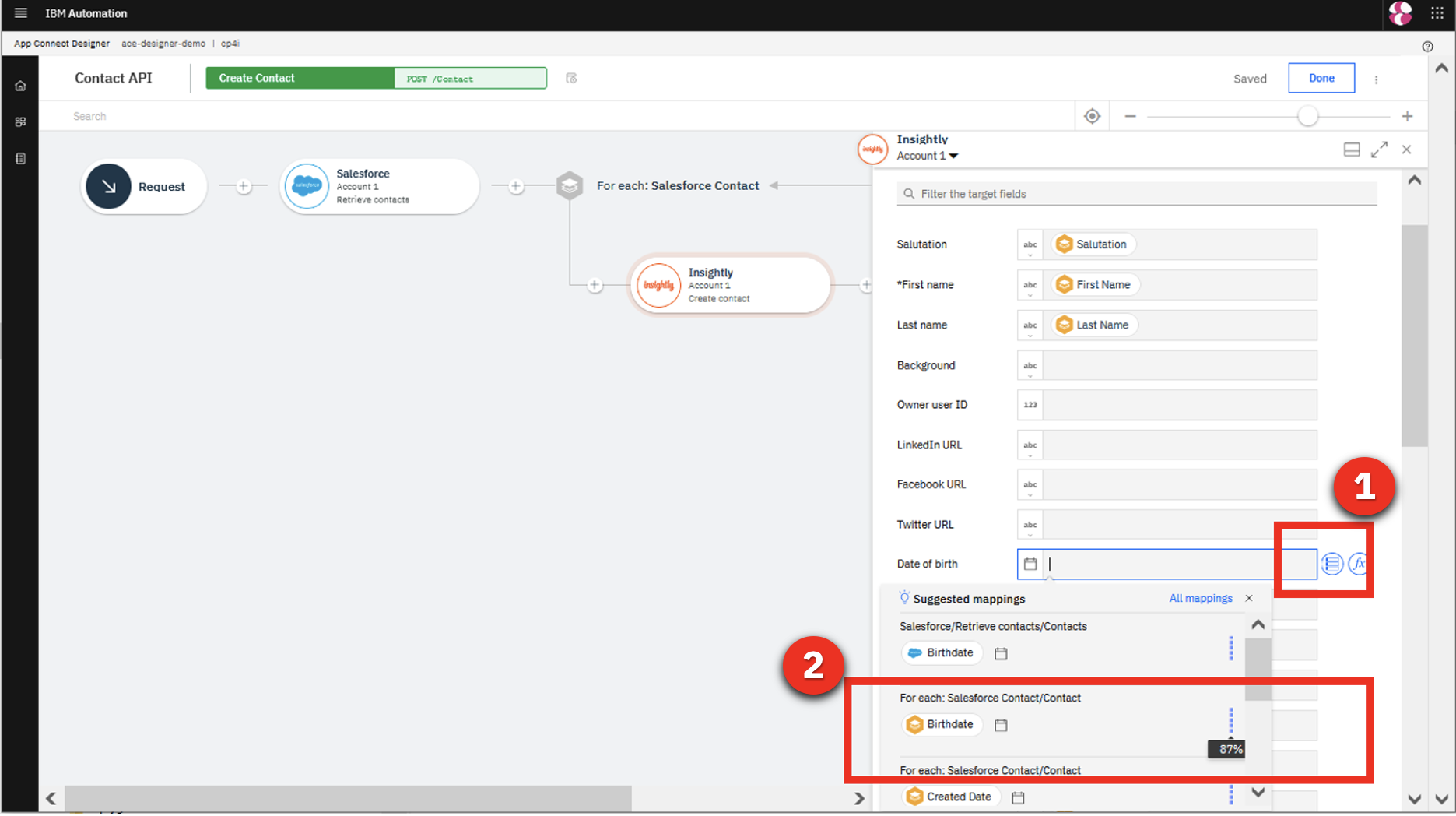
Let’s explore it with the ‘Assistant’s Name’ field. Let’s select “Generate transformation”. The ‘Generate transformation’ panel opens with five blank sources and corresponding target fields that you can use for mapping data formats. We’ll need to provide at least five examples of source and target formats for Cloud Pak for Integration to generate the transformation formula.
Using Cloud Pak for Integration and the Mapping Assist capability, we were able to easily create a data sync between two CRM solutions without needing to write any code.
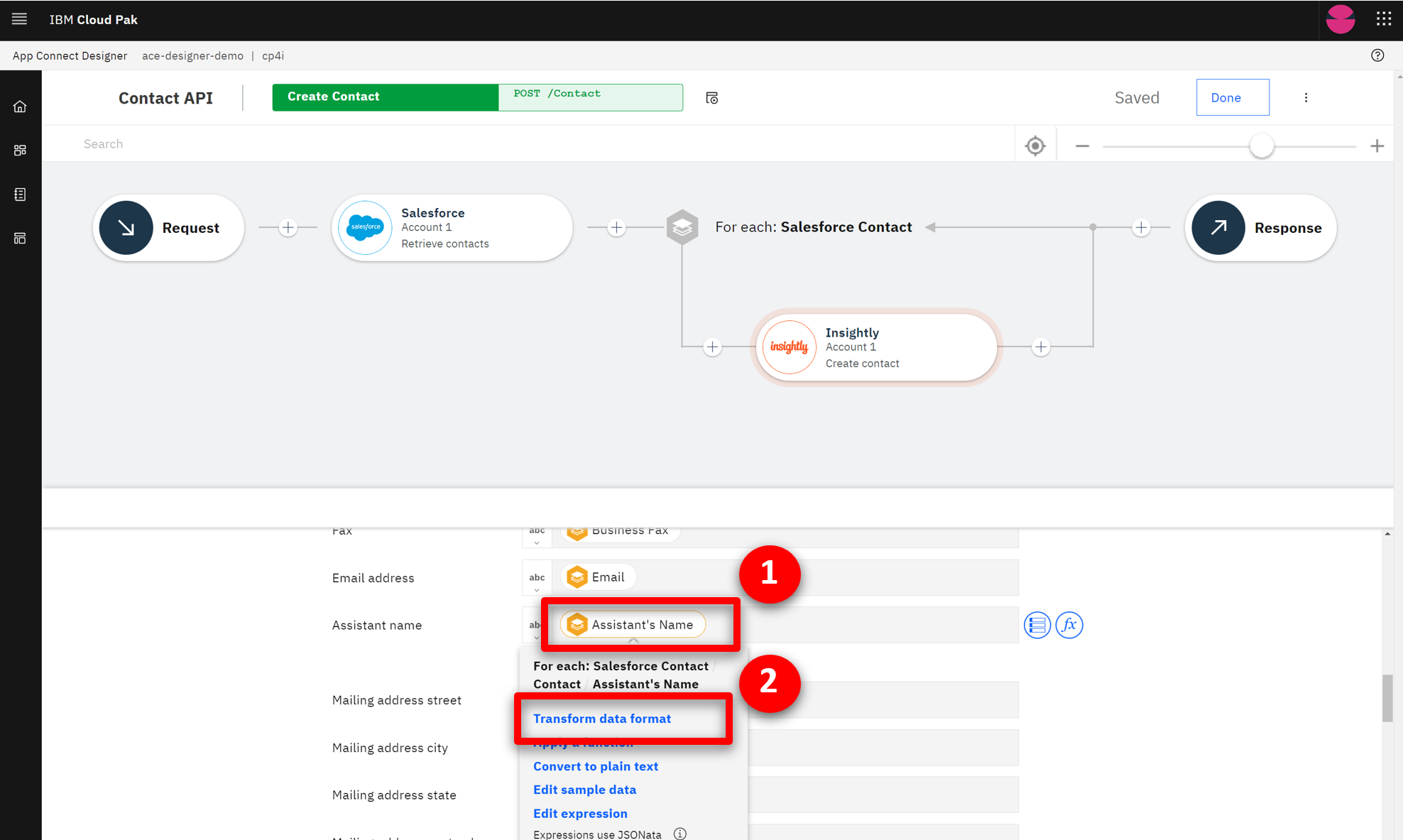
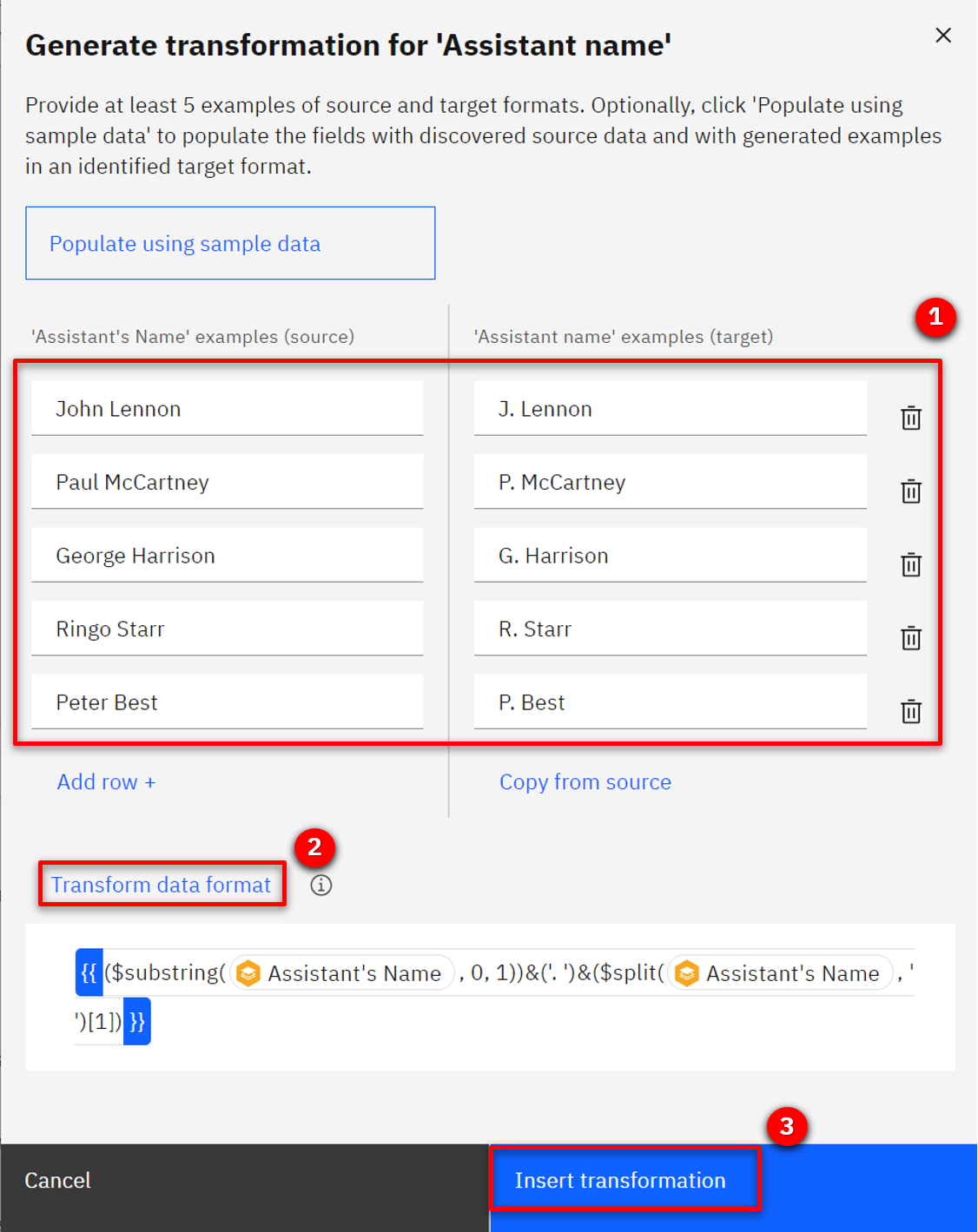
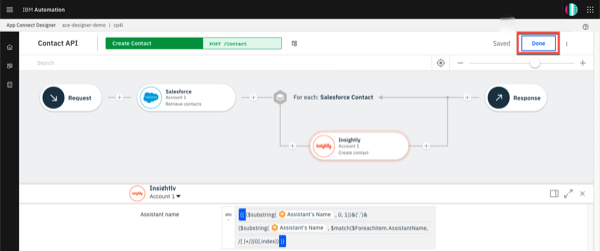
5 - Testing your data sync
Open the Contacts view (2).
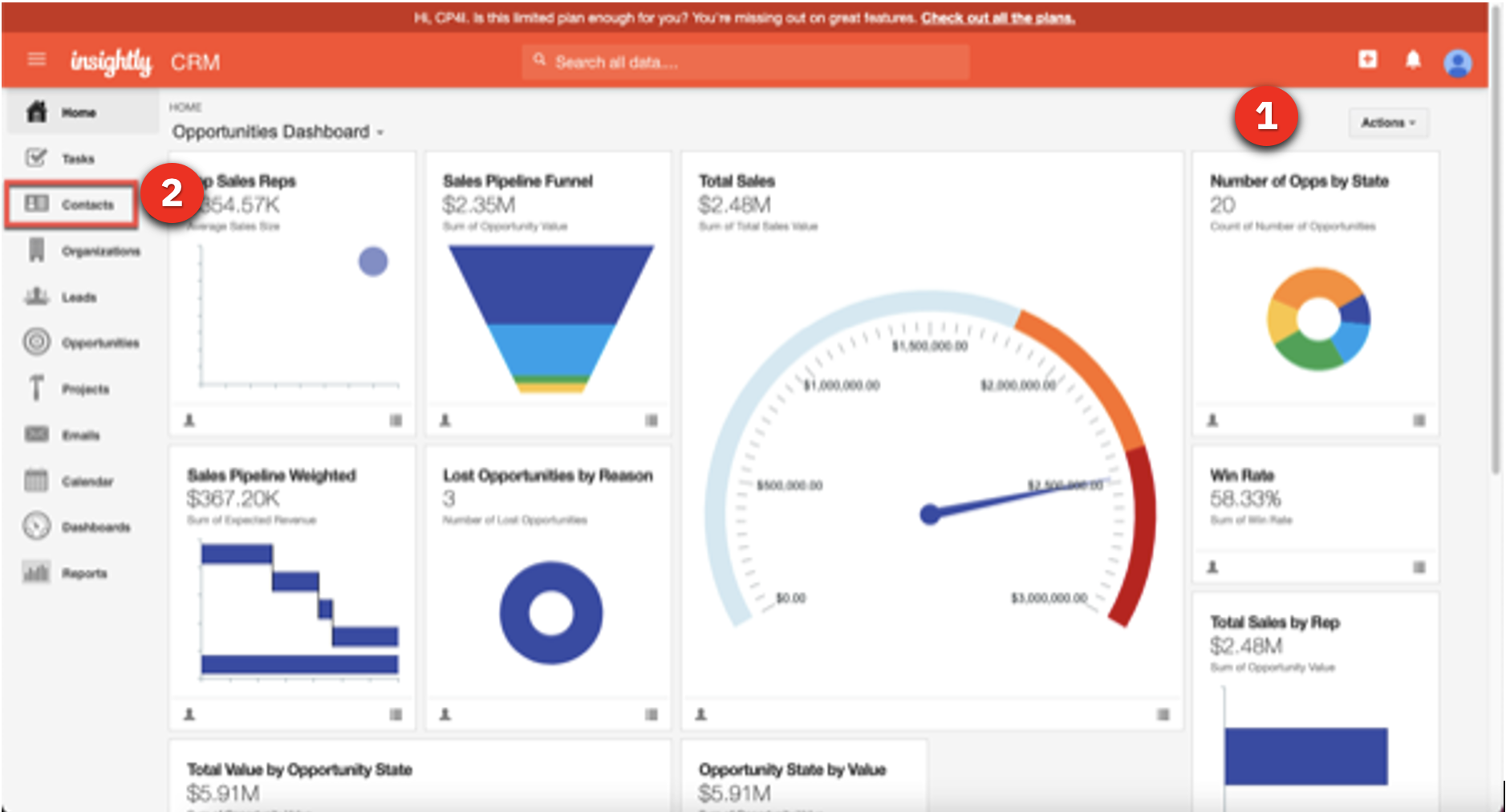
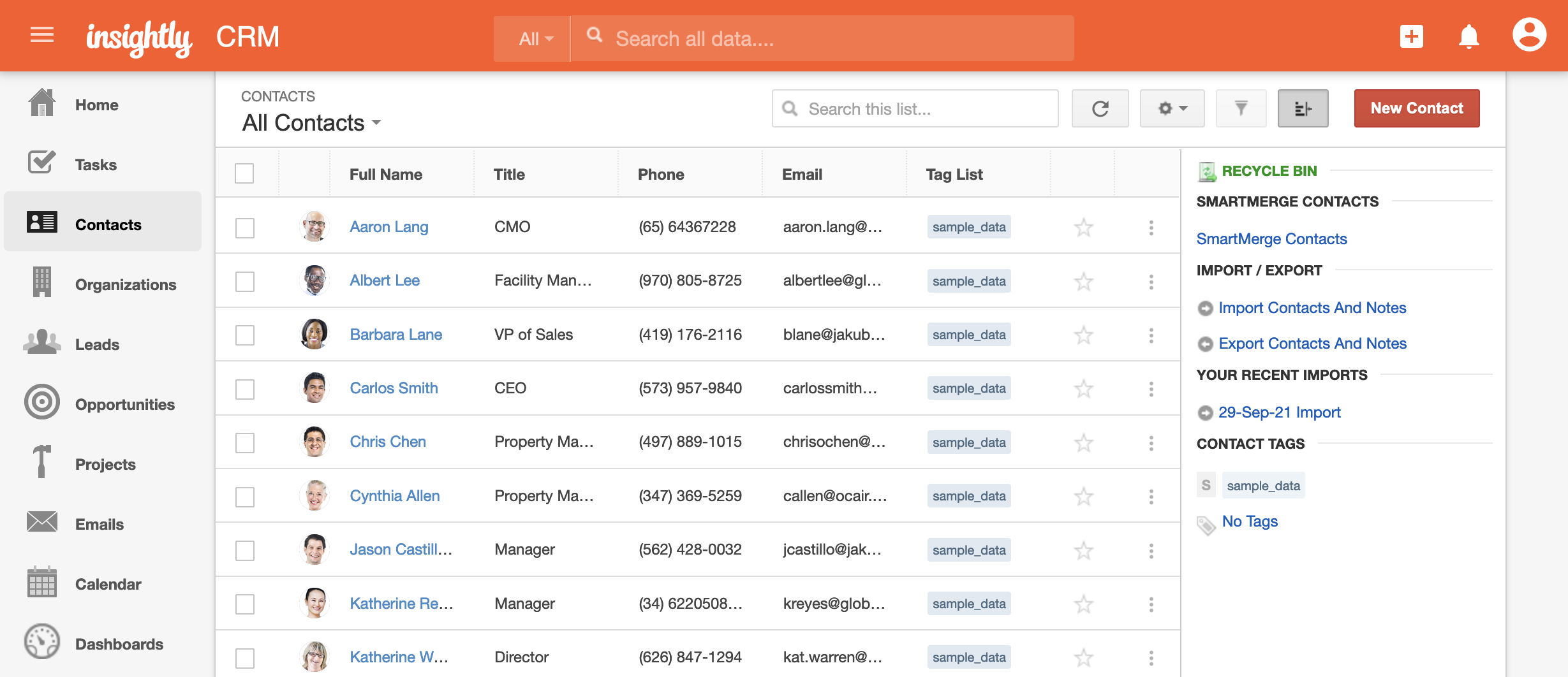
Great, our data sync is running.
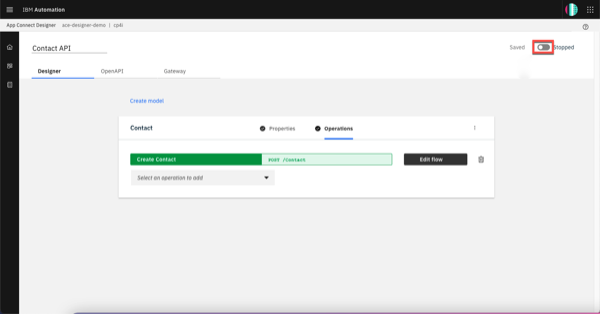
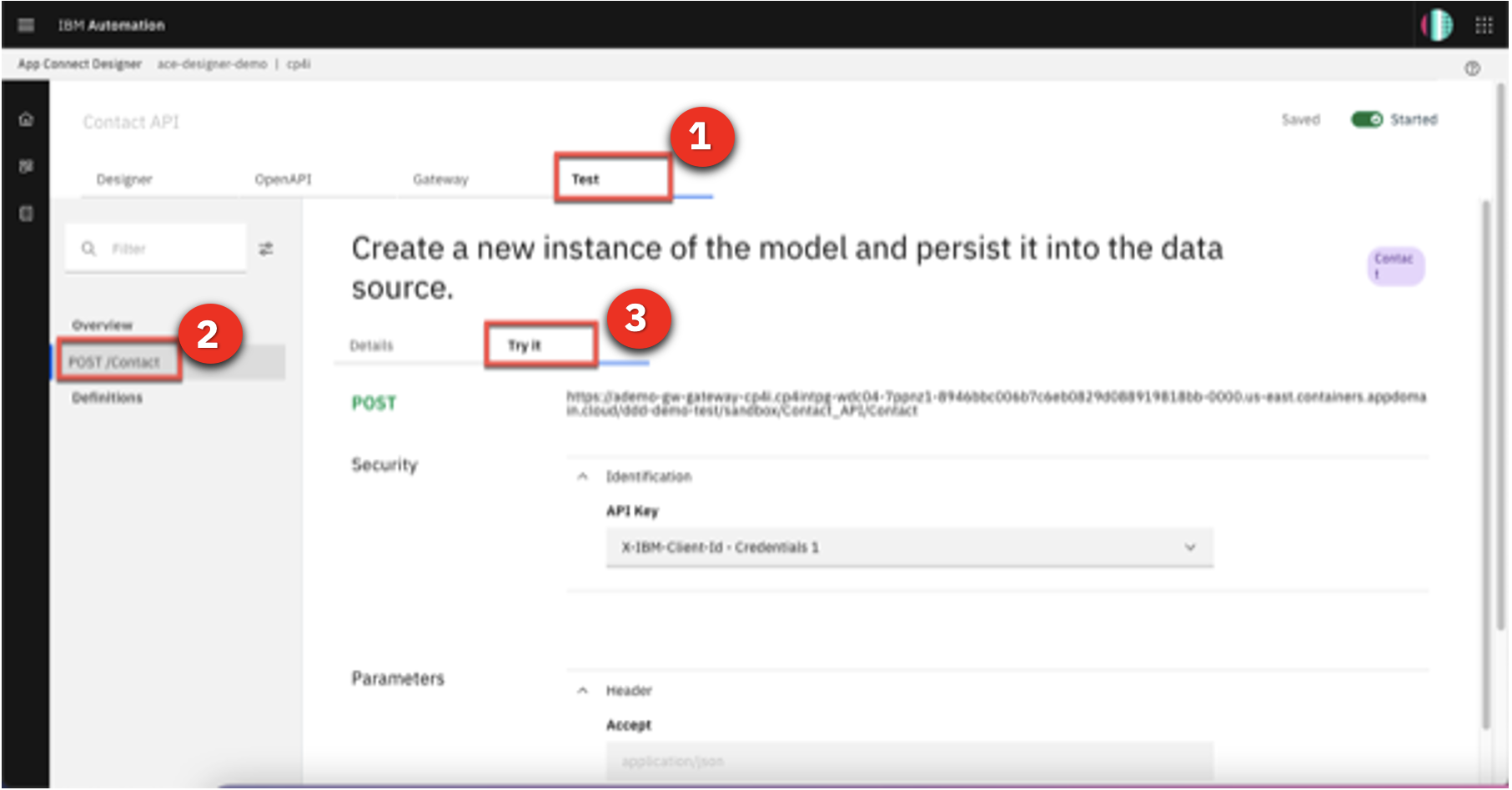
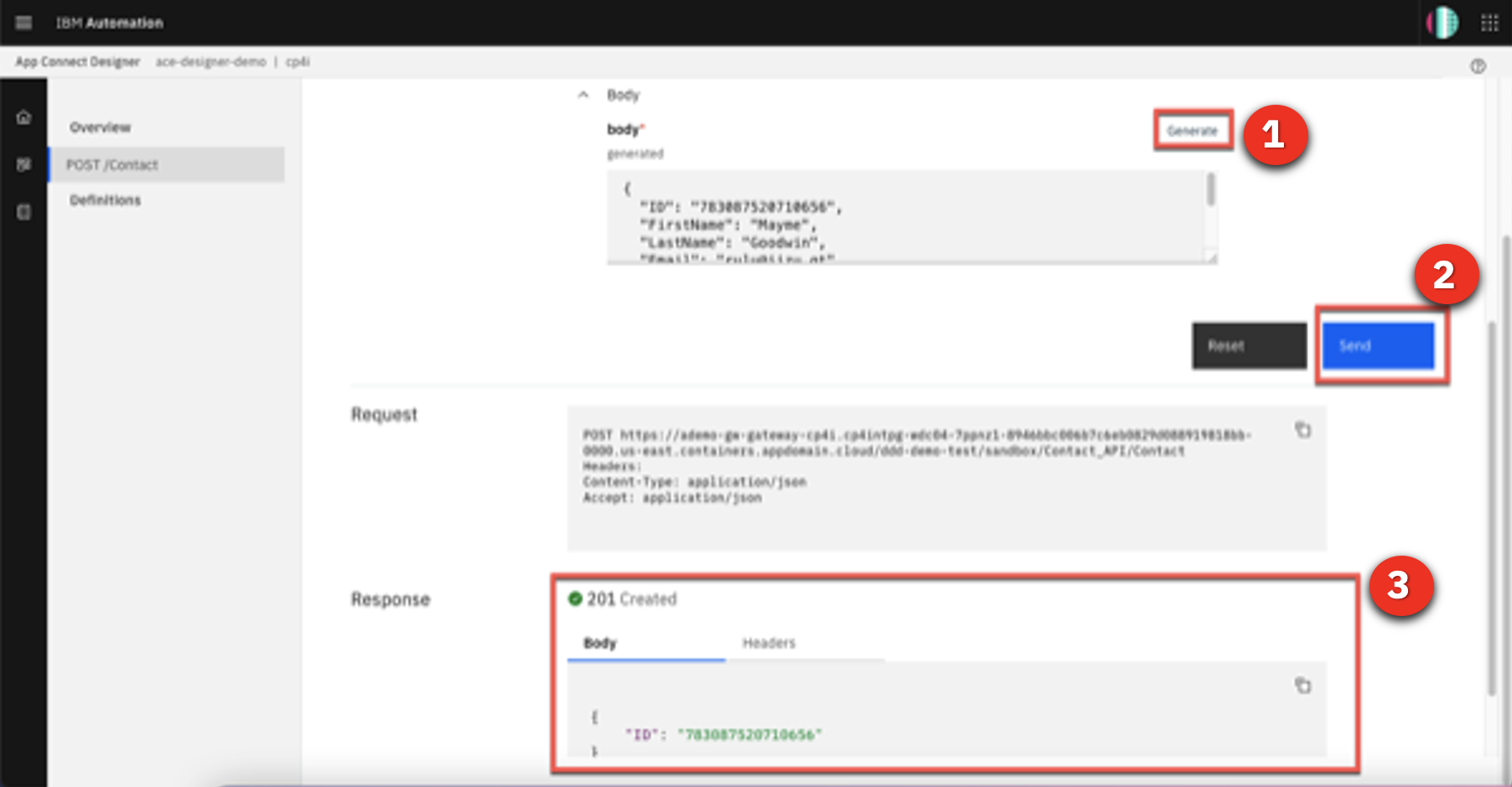
Great! Everything works as expected.
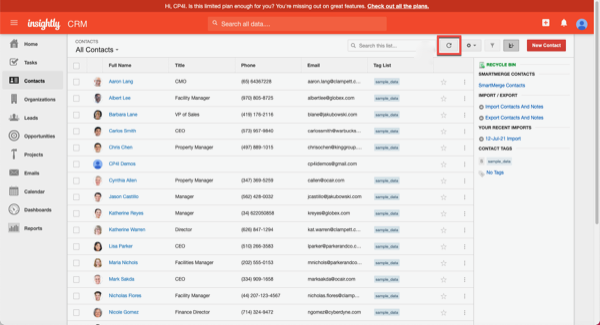
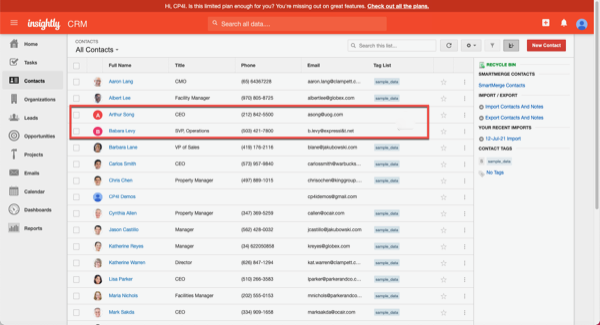
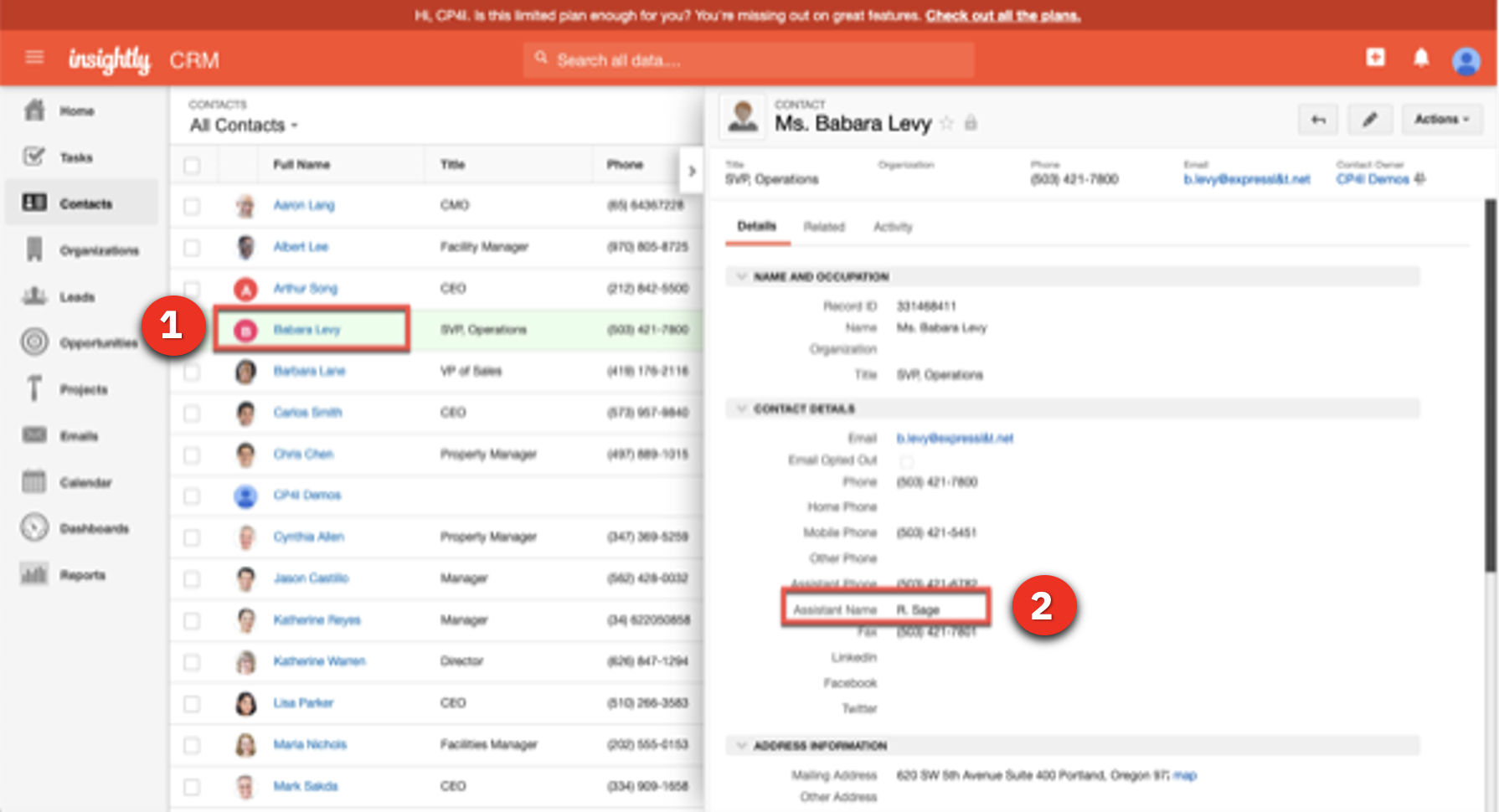
Summary
As we mentioned in the beginning, data mapping is difficult, time-consuming, and error-prone. In this demo, we showed how AI-powered Mapping Assist can alleviate these issues.
We auto-mapped all the fields where the matching confidence was at least 80%. Mapping Assist learns from your decisions about selected mappings, and shows these as top suggestions when a similar source and target mapping is attempted in the future – further reducing your mapping efforts.
We also generated a data transformation by providing examples of source and target data when fields do not have the same format. AI was used to determine the pattern and generate the transformation.
Using AI-powered Mapping Assist reduced the development time and eliminated errors as ACME Retail successfully integrated the new customer contacts from their business acquisition.
Thank you for attending this presentation.